Texifter is pioneering the use of machine-learning methods to harvest essential information from unstructured social media data. For example, Twitter feeds can generate top line and bottom line growth. This requires a text analysis tool that moves beyond simply displaying information. To do this right, the tools need to become more intelligent as users to interact with data. DiscoverText is engineered to harvest large amounts of unstructured social media data to gain insights into potential business, which fosters the creation of new strategies to drive value and insights.
Social media proliferation means that people who do not use Facebook, Twitter, or LinkedIn are now in the minority. According to “Socialnomics” author, Erik Qualman, using social media is no longer of question of yes or no, but of how well it is used. Text analysis platforms are essential in business strategies, increasingly looked to by businesses as a way to generate new revenue. 
Recently, Texifter analysts have started to use DiscoverText as a lead generation tool, attempting to find potential customers on social media channels. In preliminary research, Texifter has been able to engineer 3 custom lead generation and 2 business insight classifiers. These classifiers were formed not only around large, visible corporations, but also smaller, more social media obtuse industries, such as legal services and survey generation. 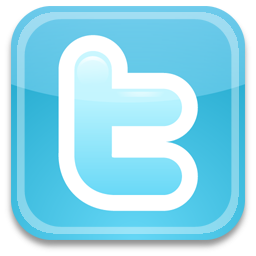
Using Twitter, analysts harvested information on one field in general, the legal profession, and 2 specific businesses, Starbucks, and McDonalds. With these archives the goal was to create a custom lead generation classifier which could be continuously refined and used over time, with the goal of identifying potential clients and business segments which could be studied further and improved upon. Whether data is big or small, DiscoverText is suited to handle the harvested text.
Legal Services
Formation of the Legal Services classifier began by harvesting Twitter for a group of “law-centric” Tweets. This yielded over 4,000 Tweets, easily enough to begin working on a
classifier. Using the coding scheme “Spam,” “Random Tweet,” and “Potential Customer,” about 400 tweets were coded, and the remainder of the set classified using the new classifier. This showed that 21% of data had the potential to be a possible legal customer, the majority of which, were often people looking for a “divorce lawyer.” This type of insight can be found by using DiscoverText’s interactive graphs, by selecting the corresponding section of the graph. Using the reply tool within DiscoverText, it is possible to respond to these Tweets immediately after discovering them. It was not shocking to find that the majority of the overall set was marred with spam, most often lawyers advertising their services, however, the classifier was very effective in segregating them when classifying. With the high amount of spam, lawyers strategy needs to change. Instead of producing large amounts of spam, lawyers should employ social media text analytics to search for their clients, instead of crowding the already noisy Twittersphere.
Real Big Data- Highly Visible, Often Mentioned Mega-Corporations
The McDonalds and Starbucks archives combined included nearly 70,000 Tweets-this coming over a couple of days, using the normal Twitter API, harvesting 1-2% of all Tweets-meaning these corporations are mentioned over 5 million of times a day. Once broken into manageable datasets, it was possible to form multiple lead generation and business insights classifiers, all specifically tailored to these large corporations business. A Starbucks dataset of over 1,000 Tweets was coded using the scheme “Potential Customer,” “Location Insight,” “Random Tweet,” and “Spam.” The classifier revealed that
46% of the Tweets were potential customers, and 18% of the data provided a location insight, meaning that over 60% of the data was valuable information. The potential customers often Tweeted about their upcoming Starbucks visit, often posing the question of what to purchase, opening the door for suggestions and given Starbucks the opportunity to promote a drink. Location insights often proved that there are many people who do not have the luxury of a Starbucks-across- from-a-Starbucks, and, that quality of service sometimes can differ depending on location- both important pieces of knowledge for any business, which can now be acted on.
Using the McDonalds data, a slightly different, “business segment” classifier was created, with the goal of developing a multi-layered classifier, one which could classify data on two levels, looking for potential leads, the coding scheme sought to identify Tweets based on different aspects of McDonald’s business, specefically with the goal of finding areas which could be improved.
Interestingly, for a “restaurant,” only 15% of the Tweets mentioned the food at McDonalds. Sure it is great to know that 15% of the Tweets are about food, but what type? Using the multi-layered classifer approach, we can create another classifier specifically tailored to just food comments, which will answer that question. When this is done, it reveals that the vast majority of Tweets do not specify a particular item at McDonalds.
However, when a particular food item was mentioned, the fries took first place. “America’s Favorite Fries,” might be working, however other brand names such as “Big Mac” and “McFlurry” might not be. Additional steps in the business insights process are endless with DiscoverText. From here, it possible to continue classifying by sentiment, or taking the individual categories again creating a new classifier.
Small Data-Survey Generation
DiscoverText recently began using the GNIP PowerTrack, taking social media lead generation and business development to another level, by giving the system the ability to ingest 50-100 times more Tweets than the regular Twitter API allowed, and much more robust metadata. This will only increase the amount of data which DiscoverText can ingest, allowing businesses to gain even more exact insight into their data, and to continuously monitor their brands on Twitter. Aiding a small survey company, we began feeds which pertained to the creation of online survey. Using the PowerTrack, more than 175,000 Tweets were harvested. but, how do we find such a specific request for survey creation help in such a large pile of Tweets?
Using just keyword searches, and DiscoverText’s Cloud Explorer, we were able to identify numerous customers who needed help generating survey participants, as well as a handful of people who needed help creating their surveys, all of which could be pursued by the survey creation company. Going out on a limb, using our built in response tool, I contacted
a Twitter user who needed help creating a survey. Using the metadata which had been harvested, I knew that his Tweet was fresh and ripe to answer. The immediate response worked, as the user acknowledged my Tweet, and was thus persuaded to check into the new survey generation site.
DiscoverText as a lead generation tool is the perfect synthesis of analysis and monitoring tools which give great advantage for business to drive value and growth online. In the future, Texifter will be posting more material on how to use DiscoverText for Lead Generation, if there is something you would like to see, contact us. Please visit the Texifter website to view our new lead generation product sheet.