[In the coming months leading up the U.S. presidential election, the Texifter blog will be featuring a multi-part series on various analytical insights into the election, which can be gained by utilizing a host of social media analytics (SMA) methods within DiscoverText. The following is the first post in this multi-part series.]
The month of April saw dramatic shifts in the landscape of the Republican presidential nomination. When the month began, Mitt Romney and Rick Santorum were still battling for support of the Republican base. And while many believed Rick Santorum’s ardent conservatism would enable him to remain in the race for quite some time, he ultimately conceded on April 10th, giving way to Romney’s presumptive Republican nomination. Meanwhile, and in spite of their inevitable loss to the Romney establishment, Newt Gingrich and Ron Paul remained in the race through April – largely on principle.
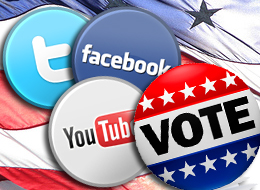
Throughout April, millions of individuals utilized social media to express their feelings about this heated Republican primary race as well as about specific Republican candidates. So as April ultimately became the month during which Romney’s nomination solidified, the question on many minds is: will Conservatives, Independents, and undecideds coalesce around the moderate Romney? Social Media provides a wealth of data from which one might begin to answer that question (which will continue to be answered in subsequent blog posts regarding sentiments, topics, and natural language processing).
For this first experiment, I kept things simple: I decided to investigate which hashtags were most commonly and most perpetually associated with “Romney” on Twitter throughout the month.
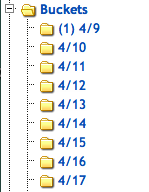
To do this, I began by setting up a live feed from the Public Twitter API in DiscoverText on April 9th. (Therefore my dataset spans from mid-day on April 9th until 11:59pm on the 30th) Once my data collection was complete, I created individual “buckets” (or saved search results) – each containing a full day’s worth of “Romney” Tweets. Next, I utilized the software’s “TopMeta Discovery” function in order to view the most frequently occurring hashtags from each day in April. I then exported each list of hashtags with the number of times each occurred per day.
Each list of hashtags-by-day contained upwards of 2,000-4,000 unique hashtags. Since my interest – for this post – is to examine the volume of the most common and most consistent hashtags, I decided to only examine the top 25 hashtags from each day. Having now brought the total number of hashtags to analyze from over 50,000 to 525, I was now left with 93 unique hashtags. Of those 93 hashtags, only 13 of them were among the most frequently used (top 25) hashtags each day. The following charts demonstrates the proportional change in volume that each of these 13 hashtags experienced in April:
[Click on any graph to enlarge image.]
All 13 Hashtags:
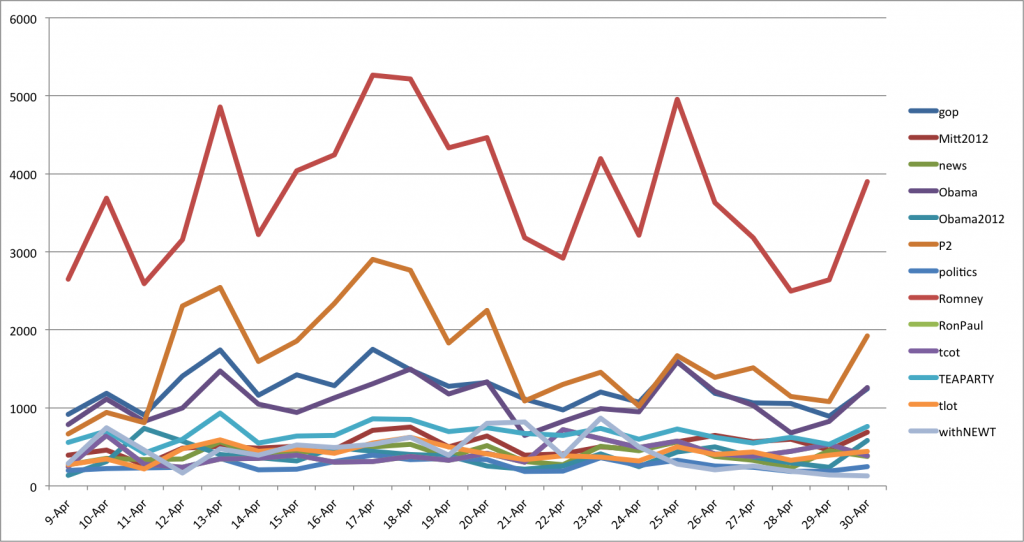
A closer look at the top 5:
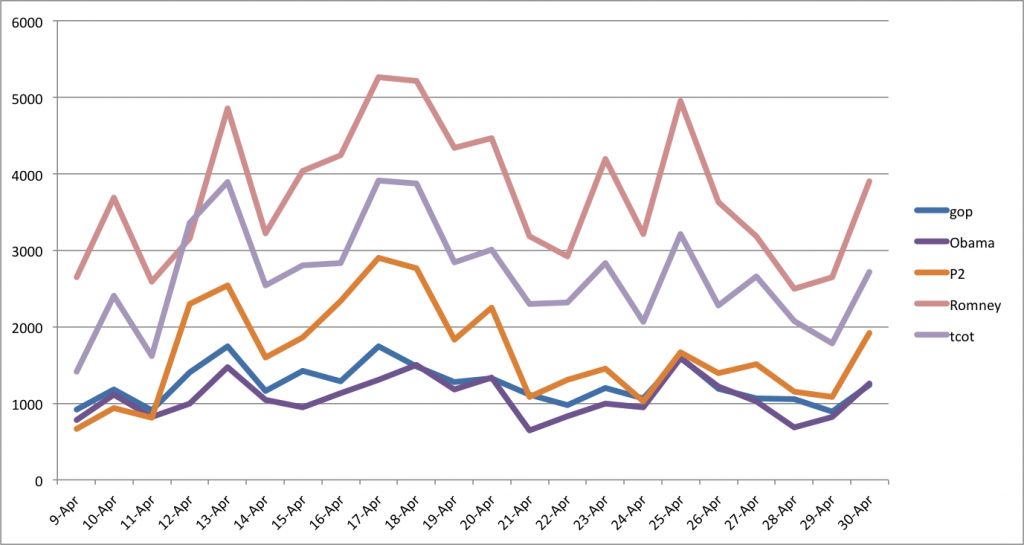
A closer look a the next 8:
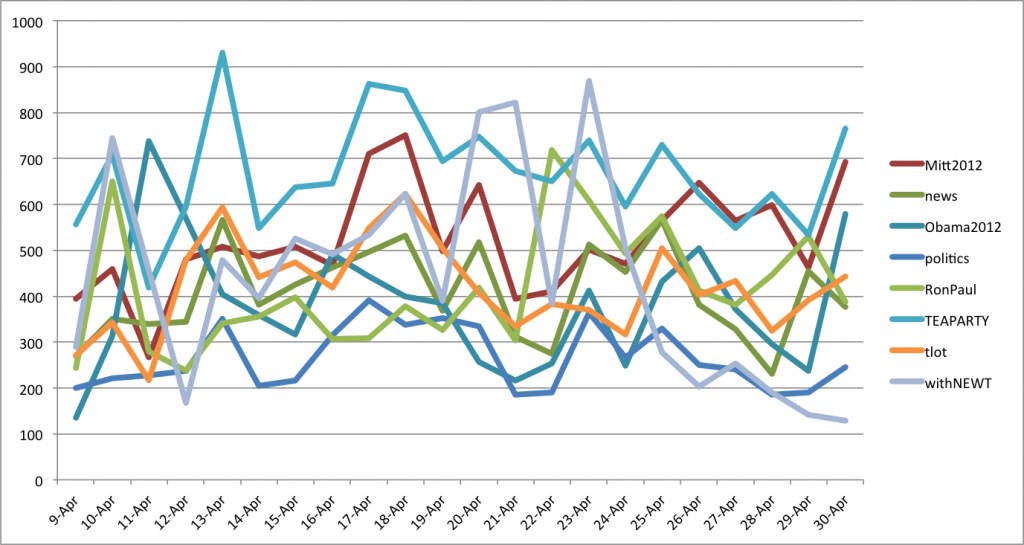
Initial Observations
With even a quick glance at the five most consistent and most common hashtags (that co-occur in Romney tweets in April), it is easy to conclude that a pattern emerges in the daily volume of “GOP” and “Obama” hashtags. The volume of one rarely deviates from the other, whereas hashtags “Romney,” “P2,” and “TCOT” differ substantially in volume while deviate in a pattern.
The daily volume of the remaining eight hashtags – on the other hand – is rather sporadic. With a cursory glance, one can plainly see the dramatic range of both “withNEWT” and “RonPaul” hashtags. Perhaps due to the concession of Rick Santorum, “withNEWT” hashtags saw an early spike in volume on the 10th, only to plummet and remain low in volume until a sudden rise and fall in volume between the 20th and the 23rd. And while “withNEWT” saw a rapid fall in volume on the 22nd, the volume of “RonPaul” tweets rapidly inclined on that same day.
Hashtags only reveal so much insight. They may provide topic (and co-occurring topic) volume, but they provide little to no true context. Until the text of these Tweets is closely analyzed, one can only speculate as to why these rapid volume shifts took place and what meaning these labels truly hold. Keep a lookout for a follow-up post about how you can use DiscoverText to uncover more nuanced context with other text analysis methods. In the meantime, if you’re interested in getting started with the software or have any questions, you can email me at Josh@discovertext.com.
Note: All tweet volume here is proportionate to the sample of tweets provided by the Public Twitter API and do not reflect the true volume of Romney Tweets. The sample volume of these “Romney” tweets are shown below:
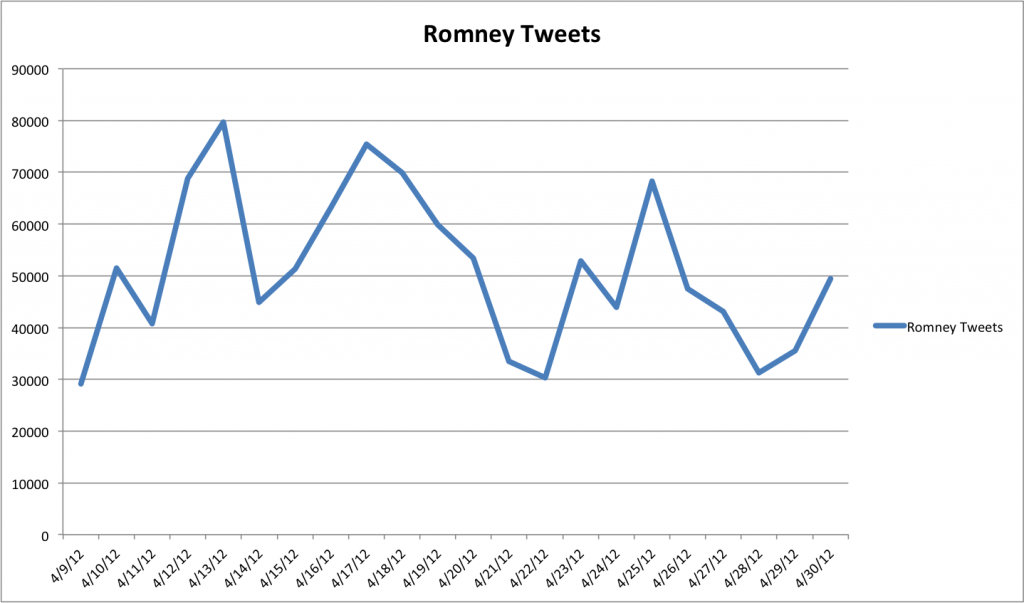